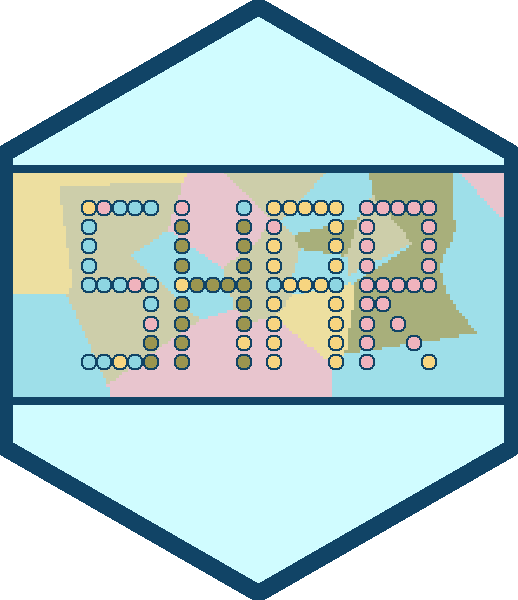
Parallelization
Maximilian H.K. Hesselbarth
2024-07-29
Source:vignettes/articles/parallelization.Rmd
parallelization.Rmd
The shar
packages has no build-in parallelization,
however, there are many R
frameworks that allow to run code
in parallel or on high-performance clusters (see e.g., future
,
clustermq
or rslurm
).
Thus, shar
provides utility functions that facilitate its
usage together with any parallelization framework.
The following examples illustrates how to use future
package to randomize patterns using fit_point_process
in
parallel using all available cores on a local machine. Similarly, the
core idea of the following code could be used to run shar
on a high performance cluster.
First, we need to load all required packages. This includes
future
and future.apply
.
The future
packages allows to run code in parallel using
only a few lines of code. By setting the future
plan to
multisession
, the package automatically resolves all
following futures
in parallel.
Importantly with this approach, you need one randomization per core
(n_random = 1
) and set simplify = TRUE
to
return the point pattern only. This results in a list of randomized
point patterns.
future::plan(multisession)
fitted_list <- future.apply::future_lapply(X = 1:39, FUN = function(i) {
shar::fit_point_process(pattern = species_b, n_random = 1,
return_input = FALSE, simplify = TRUE, verbose = FALSE)
}, future.seed = 42)
Next, you can use the list_to_randomized()
function to
convert this list of randomized pattern to a rd_pat
object
that will work will all other functions of the shar
package.
fitted_rd <- list_to_randomized(list = fitted_list, observed = shar::species_b)
Lastly, the created objects can be used to analyse if species-habitat associations are present as usual.
landscape_classified <- classify_habitats(raster = terra::rast(landscape), n = 5, style = "fisher")
results_habitat_association(pattern = fitted_rd, raster = landscape_classified)
#> > Input: randomized pattern
#> > Quantile thresholds: negative < 0.025 || positive > 0.975
#> habitat breaks count lo hi significance
#> 1 1 NA 6 14.90 29.15 negative
#> 2 2 NA 18 26.95 49.05 negative
#> 3 3 NA 18 21.65 43.15 negative
#> 4 4 NA 21 27.00 52.15 negative
#> 5 5 NA 129 54.95 74.10 positive
Of course, this idea can be used to randomize the raster data as well. Furthermore, any other parallelization framework could be used.